In most organisations of today you collect large amounts of data from within and outside of the organisation for various purposes. It is everything from financial information to information from various sensors in equipment sold or purchased and sometimes also personal data from individuals that you either do business with or wants to do business with. This applies no matter if you are in the B2C (Business to Consumer) or B2B (Business to Business) space.
Treated and analyzed correctly all this information can give your organisation very valuable insights and this is of course what this whole data & analytics readiness is about, but at the same time as using data can give you many benefits it also introduces some new risks. There are laws and regulations (for instance GDPR) that affect how you are allowed to share and use certain kinds of information. There are also moral aspects to how you can use personal data to analyze the behaviour of individuals or groups of people.
These considerations where often made in relation to the initial decision to gather specific data, but when using them for analytics it might be that these data are utilised in new ways and maybe also cross-referenced with other data that can call for new considerations into these aspects. It is also important that new use of gathered data does not compromise security, so that individuals or organisations that was never intended to gain access to this information will do so if they are used in a new context.
This is why it is important to also consider who has access to the data when used in new ways.
Five considerations
To cater for all these concerns there a number of things that you need to do, or at least take into consideration, when launching a Data driven initiative within your organisation. Here is a list of some of these considerations:
- Laws and regulations
Make sure that you are not violating and laws or regulations when using data for analytics. We can use GDPR as an example in this case. You need to assure that the processing and/or use of personal data is not violating and consents given by individuals when collecting the data or that data is distributed in a way that someone who is not intended to have access to specific personal data gains such access. This can for instance be managed by anonymizing or aggregating the data so that individuals can not be easily identified. Ask the data owner if you are insecure and it is not clear how you can use the data. Much useful information on various kinds of behaviours can still be drawn from aggregated data.
- User access management and secure password management
Aggregation of data and collection of data from various systems can be a great opportunity to do advanced analysis, but it can of course also pose a risk that data is accessed by individuals or systems that were never intended to have this access. It is also potentially a great risk if someone illegally gains access to this data, as it can potentially reveal business or trade secrets. The aspect of user access management and secure access to these applications and systems are therefore of great importance. Make sure to implement routines to protect this data already from the start.
- Secure connections
Another area of increasing concern when it comes to IT security these days is the growing number of system to system communication without human interaction (often by using APIs). At the same time as this of course creates opportunities it also creates a challenge to limit access to only those systems and queries that are supposed to have access. It might also be that certain systems should only have access to subsets of data and not the entire load of data. It is also a fact that APIs are becoming the primary attack vector for illful hackers that are trying to gain access organisations data. This is why it is important to have a scheme for how to protect potential APIs that gains access to your data already from the beginning and keep these routines updated.
- Encryption
Another way of protecting your data from illful usage, is to encrypt the information. This is a good way to make sure that even if someone should gain access to the information, they will not be able to use or interpret it. However, this comes with some overhead in terms of work and tooling required to both encrypt and decrypt the data whenever you want to use it yourself (or your organisation). Therefore this is a consideration that will require some initial thinking before being implemented.
- Version control of data products and documentation
As mentioned in previous posts, analytical models and data products can be run several times on the same data set to gain new insights or to improve a specific analysis. It might also be that the model is used for new sets of data. In both cases it is important to keep track of which version of a specific function that has been used for the analysis and what set of data that is used. This is why it is important to keep track of versioning and documentation related to these data products. For instance it might be that one specific analysis works terrific and you want to re-use for new data sets, then you don’t want to loose this specific version of the data product. Another possible use case is that the data product produces a faulty result for some reason. Then you would like to trace this data product to correct the issue or avoid to re-use this version for new analysis.
The above listed bullets are just examples of considerations that you need to do related to security and governance for your Data driven initiative. What you really should aim for in your initiative is to create an understanding for the importance of security and governance to protect data used for analysis. Rather than creating a specific role or dedicate a person who is responsible for this area, you should aim to establish a ”Way of Working” that incorporates these considerations automatically in day-to-day operations and development.
This can be supported by guidelines and simple routines so that developers/data scientist/data engineers etc will know how to act to protect the interests of your organisation when it comes to smart usage of collected data. To our experience this is the most efficient and smartest way to ensure that data is protected and governed, while still able to be utilised for your Data driven initiative and business development.
More information
Redpill Linpro is launching our Data driven ready model in a viral way by releasing a series of blog posts to introduce each step in the model. This is the fifth post in this series. Below you will find a ”sneak-peak” into the different steps of the model. Stay tuned in this forum for more information on how to assure Data driven readiness...
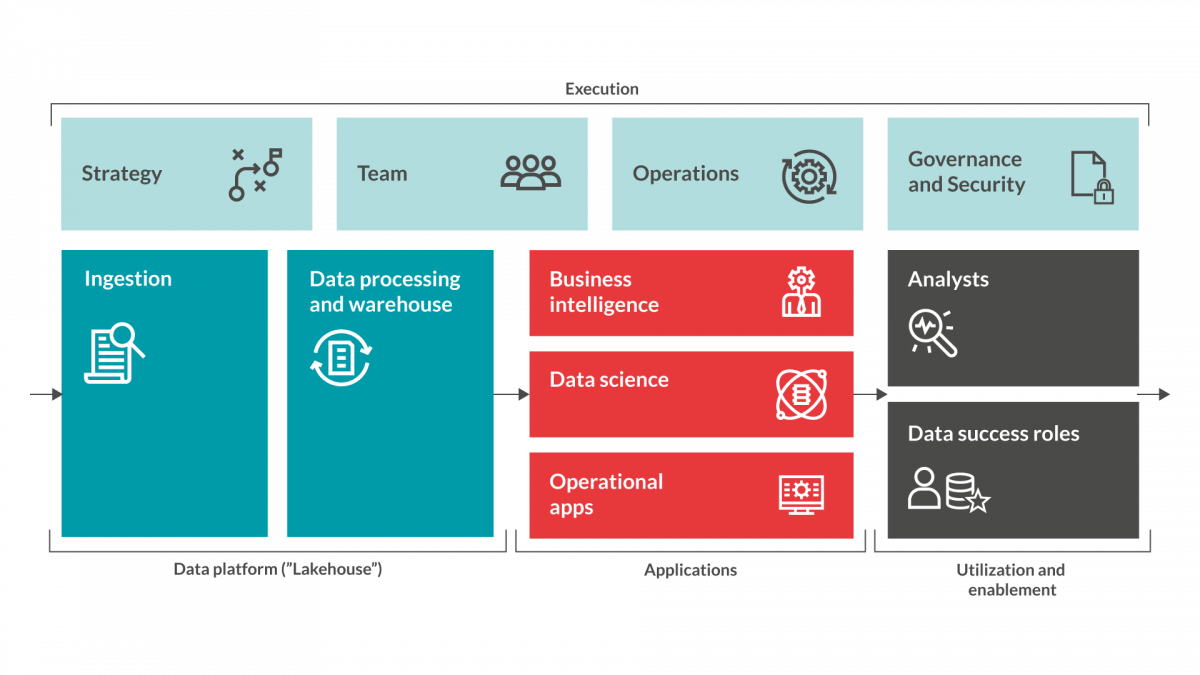
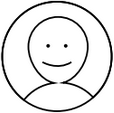