We call these activities for ”Applications” of data, as the output is where data, metrics and insights are being consumed and applied after having been made available from the Data processing and warehouse platform (see step 6 in the Data driven ready model).
With the ”Applications” of the model we will try to structure the actual output of the data & analytics work into three different categories: Business Intelligence, Data science and Operational applications.
Business Intelligence applications
The first kind of applications that we will dig a bit deeper into are Business Intelligence applications. These applications are often used to make data readily accessible to the organisation and its processes. This means that when developing these applications, it is often required to work closely with business users, analysts and the data engineers.
The business intelligence applications refine the data available and provide the organisation with dashboards and reports based on requirements from the business. The purpose is to provide self service Business Intelligence capabilities to the organisation (i e to make it possible for business users to ”drill down” into reports and data to gain business insights based on data available) and business insights. Business intelligence applications makes use of data available from transactions and activities that has already occured.
This means that Business Intelligence is focused on analysis of past data and things that has already happened. This provides valuable business insights into how various decisions, patterns, pricing, marketing activities or other events have affected the business. However, it does not focus on prediction and models to support future decision making.
Data Science applications
The Data Science applications on the other hand are more focused on machine learning/AI, advanced analytics and predictive analysis. Roles like Data Scientists, Machine Learning Engineers and Advanced analytics work with prediction models to predict or forecast KPIs like churn (customer loyalty) and lifetime value prediction (i e the total lifetime value of a certain customer engagement/relation based on a number of facts and investments in for example marketing).
If these models are successful, they can of course provide valuable information to management on how to prioritize activities like marketing and sales, but also which measures that can be taken to improve customer loyalty. All items that can affect business profitability significantly. Data Science applications can further be used to work with classification models like customer personas or other conditions that will affect which measures the business can take to attract and reatin customers.
Data science applications can also be utilised to do what is often referred to as A/B testing. Basically this means that you use data models to test possible scenarios based on various input, like ”is the new version better than the old?” Using data models this can be tested and predicted without having to do changes in real life and await the result of the action, which of course makes it a lot easier to make the right decision on whether to implement or not. This way Data Science can be used for predictive analytics and decision support.
Operational applications
The last category of applications within data analytics are the Operational applications. These are sort of the ”Other applications” that can be fed with data when it is accessible and processed in a data platform/warehouse. When data is available, it can be utilised by these operational applications to package data in a way that can be used in other business applications.
A few examples of this are marketing automation platforms, customer support, accounting systems, data products, CRM/automated marketing platforms and so forth. Basically the Operational applications feed the above mentioned applications with data sets that has been ready-made to suit the formats available in these applications.
The applications are often owned by other parts of the organisation and the output of the data analytics operational applications can be seen as a service offered based on the ability of the data platform to structure data in a usable way.
More information
In our upcoming blog post on the Data-driven ready model, we will focus on utilization of the data and enablement of the organization to make informed decisions and use the data and applications mentioned in this blog post to become truly data driven.
Redpill Linpro is launching our Data driven ready model in a viral way by releasing a series of blog posts to introduce each step in the model. This is the eighth post in this series. Below you will find a ”sneak-peak” into the different steps of the model. Stay tuned in this forum for more information on how to assure Data driven readiness....
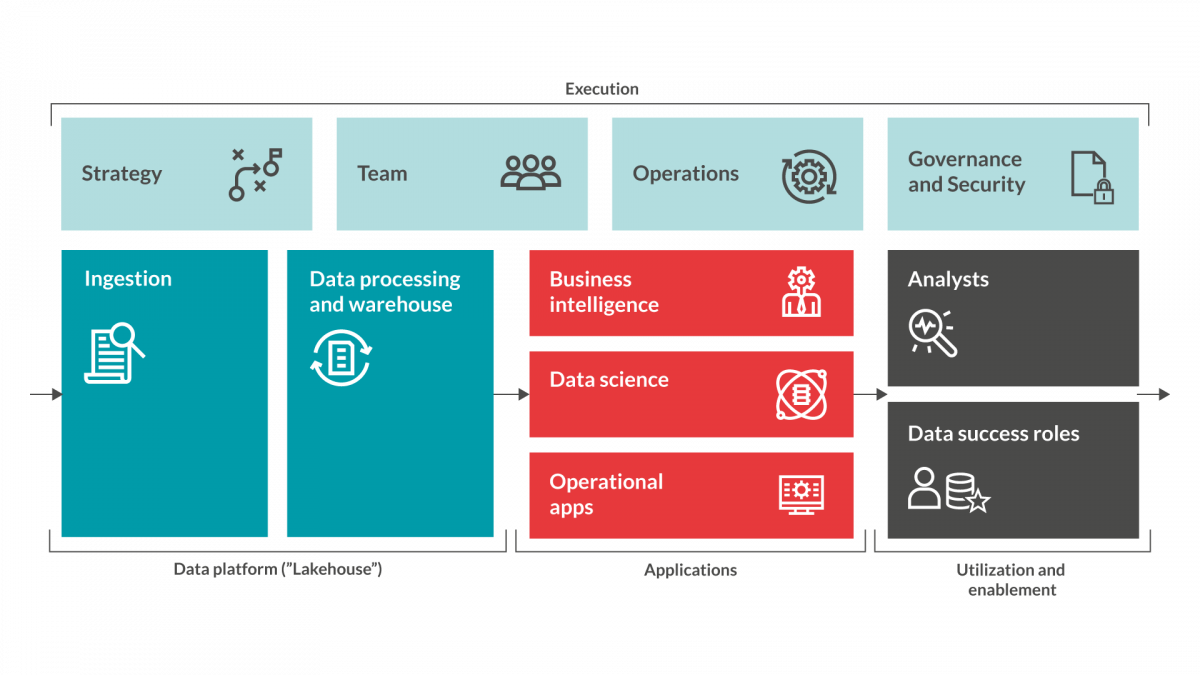
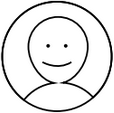