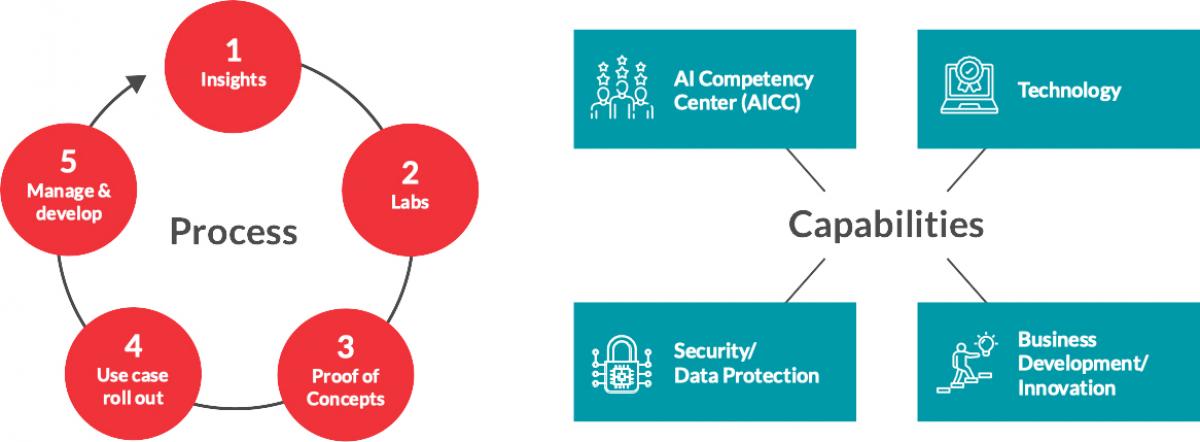
While this phase is not exclusive to AI use cases, it represents a fundamental process for the implementation and management of systems and applications. In today’s rapidly evolving and innovative landscape, organizations often overlook the comprehensive responsibility for the long-term development and resilience of newly deployed technologies.
This oversight can result in challenges with achieving the full potential of the applications or models, as well as pose security risks if they are not properly managed and updated regularly.
Ensure conditions for sharing data
To avoid these risks, it is just as important as with any implemented system or application, that you also establish these routines for your AI use cases. If you work for a larger organisation, there are probably already generic routines for this in place that can be adapted to the AI use cases. Specific things that you need to take into consideration for AI models or applications is to ensure that conditions for sharing data doesn’t change for the underlying models that you use. You also need to ensure that license terms or conditions change in a way that affects the way data is managed or used. For certain AI use cases, you also need to continouosly verify that the model performs in a way that is consistent with the expectation and process it was originally implemented for. Using AI robots or agents to perform tasks that was originally intended for humans, creates new requirements for monitoring and maintenance of these agents in a way that corresponds to how these processes were monitored when humans managed them. This blog post can not cover all these use cases, or detail how these should be monitored or managed, but rather pin-point the need for making sure that you establish systems that monitor these in other ways than you are used to for todays applications.
Key factors to consider
Ideally your organisation will look into how to maintain, monitor and develop your AI use cases. One unit can potentially be given the task of establishing these routines is the AICC (AI Competency Center), that will be covered in coming blog posts. If you have not yet established an AICC, you might have to make sure that the project assumes the responsibility also for the further maintenance of the AI implementation until the rest of the organisation is prepared to take over.
During the planning and implementation phases of the project, it is crucial to consider several key factors. An integral part of this process involves actively engaging with and educating the operations, maintenance, and DevOps teams about the specific AI use cases and models being implemented. By doing so, the organisation can effectively manage these AI initiatives, ensuring they align with existing structures while integrating the unique aspects of AI. This educational approach not only facilitates smoother management but also promotes broader AI adoption across the organisation.
Closing the circle
With this blog post, we close the circle on the process part of the AI use case implementation phase. For coming use cases you simply start all over with the Labs/Proof-of-Concept phases described in earlier blog posts (these phases can of course be run in parallell).
More information
In coming blog posts we will describe the capabilities and functions that we believe will be important to support your AI use case implementations and further maintenance. We will cover these coming blog posts of this AI Ready series introducing the ”Redpill Linpro AI Ready Model”.
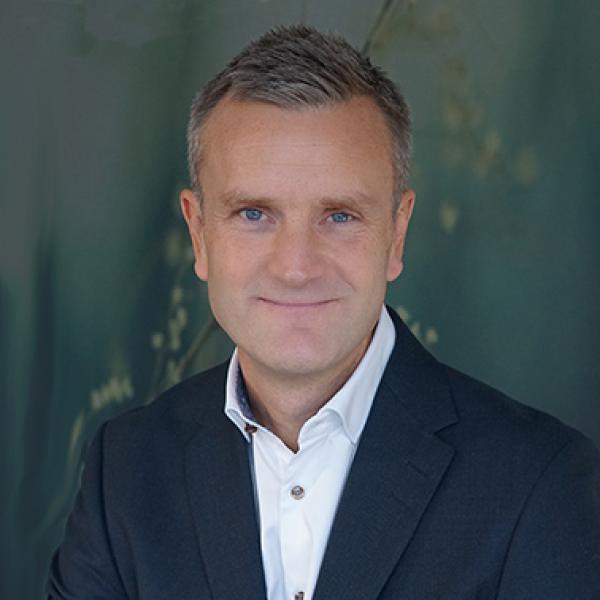
Talk to us
Fredrik Svensson
Chief Business Development Officer
+46 70 603 36 35
Why talk to Fredrik?
With decades of experience in the IT industry, he can guide you through important topics such as developing effective exit strategies, exploring European alternatives for cloud services, assessing your organisation’s readiness for AI, and understanding best practices for APIs and integrations.
This knowledge empowers you to make informed decisions and strengthen your digital strategy.
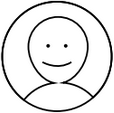